Görüntü i̇şleme ve derin öğrenme teknikleriyle çay bitkisinde fitofag akar kaynaklı hasarın tespit edilmesi
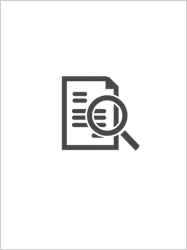
View/ Open
Access
info:eu-repo/semantics/closedAccessDate
2024Author
Tören, MuratAkıner, Muhammet Mustafa
Kaba, Bengisu
Yazıcı, Feyza
Asan, Berkutay
Beriş, Fatih Şaban
Metadata
Show full item recordCitation
Tören, M., Akiner, M., Kaba, B., Yazici, F., Asan, B., & Beriş, F. (2024). Detection of Phytophagous Mite Induced Damage in Tea Plant by Image Processing and Deep Learning Techniques. In 2024 8th International Artificial Intelligence and Data Processing Symposium (IDAP) (pp. 1–8). IEEE. https://doi.org/10.1109/idap64064.2024.10711152Abstract
The objective of this study is to ascertain the structural variations resulting from the detrimental impact of invertebrates on tea plant leaves. To this end, image processing and deep learning techniques were employed to identify plot regions in Artvin, Rize and Trabzon, where tea cultivation is highly prevalent. The use of drones enabled the acquisition of images in these regions. The results of the examinations indicated the presence of tea pest mites in the region in question. As of recent years, studies were initiated to determine the damage observed in the region, following the commencement of image acquisition. The damage was identified with an accuracy rate of 98% through the utilisation of the YoloV8 algorithm, which represents a sophisticated image processing technology, in conjunction with the convolutional neural network deep learning method. This was achieved through the application of 70 training periods to 800 images. The Edge Step (ST) method was employed to ascertain the geometric differences in the tea leaves, while the Convolutional Neural Network (CNN) was utilised to achieve the minimum classification error. The images were captured using a UAV specifically designed for the study, as it offered a convenient solution even in challenging terrain. The images were subjected to RGB, HSV and black and white colour transformations through the application of image processing techniques. Subsequently, the images were resized and divided into two classes, namely healthy and diseased. The utilisation of these data sets, divided into two classes, facilitated the attainment of more expeditious and precise results through the deployment of the image detection algorithm YOLOv8I-seg. Consequently, the rate of damage propagation was also expressed as a percentage. The study has facilitated the early detection of this damage, which represents a significant threat to tea yield. This has enabled the presentation of valuable data sets that can inform strategies for preventing a decline in yield and enhancing tea quality. These data sets provide an early warning system for combating the harmful organisms that affect the tea plant, which is the region's primary source of income.